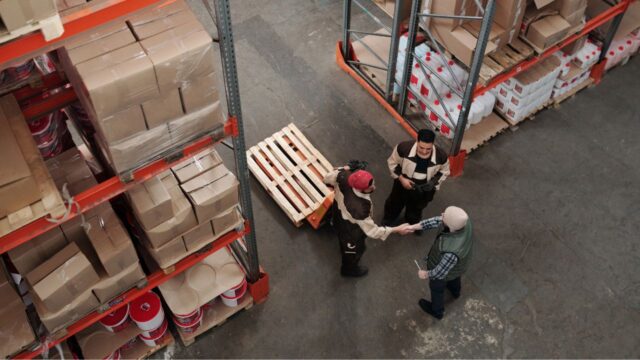
Nonparametric statistics are based on distributions that are non-normal, which means they don’t make assumptions about sample size or sample distribution. In addition, nonparametric statistics are more robust, making them an ideal choice for scientific analysis. Let’s look at some common uses of nonparametric statistics. In financial analysis, for example, a financial analyst might want to estimate the value-at-risk (VaR) of a particular investment. To do so, he gathers data from 100’s of similar investments over a similar time period. The analyst does not assume that earnings will follow a normal distribution, but rather estimates the distribution nonparametrically. In other words, the nonparametric estimate of VaR is based on the 5th percentile of a histogram of the earnings of similar investments.
Nonparametric statistics are not a normal distribution
The term “nonparametric statistics” refers to statistical methods that do not make any assumptions about the underlying distribution of data. This type of analysis is used to find out the shape of the data rather than the individual parameters of the distribution. Because nonparametric statistics are not based on a normal distribution, their parameters are flexible and can be estimated from the observed data. The following paragraphs describe some common nonparametric statistics.
Amongst the most common nonparametric tests are the Kolmogorov-Smirnov and Anderson-Darling tests. These tests compare the observed data to the quantiles of a given distribution. They are appropriate when the sample size is small. The hypothesis for this example is given below. At 5% level of significance, the hypothesis is a statistically significant. If you have a sample size of less than ten, you should use the nonparametric test.
The term “nonparametric” comes from a Scottish physician and mathematician, John Arbuthnott. He was one of the first to use the term “nonparametric” and started referring to nonparametric statistics in 1942. In 1945, Frank Wilcoxon introduced the first nonparametric method – rank – as a means to compare groups of different sizes. This technique was further developed by Henry Mann, who used it in comparisons of groups of varying sizes.
They do not make assumptions about sample size
Nonparametric statistics do not make assumptions about the sample size. For example, the t-test is robust to the assumptions about normality and equivariance. This means that nonparametric statistics are more appropriate for data that are skewed, rank-ordered, or discrete. Since they do not make assumptions about sample size, nonparametric tests have lower sensitivity and less information inherent in the assumptions. However, larger samples mitigate these limitations.
The main differences between parametric and nonparametric statistics are in the way they handle data. The former relies on the differences between group mean and median values, while the latter emphasizes rank and size of the groups. Nonparametric statistics are often used in exploratory studies, and their results can be misleading. However, there are ways to improve the quality of these results. To learn more about nonparametric statistics, read the following explanation.
Because nonparametric statistics do not make assumptions about sample sizes, they are not as precise as parametric statistics. For example, the Mann-Whitney U-test tests the difference between two samples’ means, while a t-test requires a computer and calculator. Despite this, it can be useful in a variety of scenarios, such as determining the size of an experimental group.
They are more robust
There are two main types of statistical tests: parametric and nonparametric. Parametric tests require specific assumptions, and nonparametric tests can be used for more flexible purposes. Nonparametric tests are robust, but are not as effective as parametric tests. They allow researchers to reach conclusions based on exact probability values or confidence intervals, which are more flexible. Nonparametric tests also have fewer drawbacks, however.
When compared to parametric tests, nonparametric tests involve much less computation. The difference between these two types of tests comes down to how many data points are available for each. If the data set is continuous, transforming it to ranks is a waste of information. In contrast, if data is ranked, a nonparametric test will not yield a falsely low result. However, this is the case only if the sample size is large.
In this study, nonparametric methods were used to analyze electrophysiological data. Similarly, nonparametric tests were used for whole animal behavior. Moreover, they were more robust when comparing data from two different groups. In this study, both the experimental and control groups had similar feeding rates. For nonparametric testing, a test statistic’s distribution is usually a close approximation of the conventional parametric distribution.