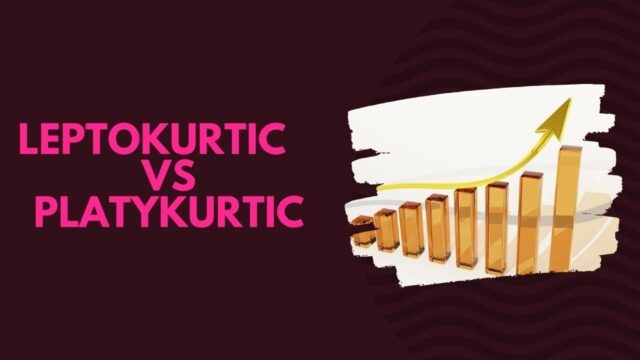
There are two types of kurtosis that data can have- leptokurtosis and platykurtosis. Leptokurtosis is a type of kurtosis where the data points are more peaked than normal, while platykurtosis is a type of kurtosis where the data points are more spread out than normal.
In this blog post, we’ll go over what leptokurtosis and platykurtosis are, how to tell if your data is leptokurtic or platykurtic, the benefits of having a leptokurtic or platykurtic dataset, how to adjust your data to make it more leptokurtic or platykurtic, when leptokurtosis or platykurtosis is a problem, examples of datasets that are either leptokurtic or platykurtic, and further reading on leptokurtosis and platykurtosis.
Additional Reading:
What is Leptokurtosis and Platykurtosis?
In statistics, kurtosis is a measure of the “tailedness” of a distribution. A distribution with heavier tails than the normal distribution is called leptokurtic, while a distribution with lighter tails is called platykurtic. The standard definition of kurtosis is based on moments, so it is not directly related to the shape of the distribution.
Instead, it describes how likely it is to observe values that are far from the mean. distributions with high kurtosis tend to have skinny tails and pointy peaks, while distributions with low kurtosis tend to have fat tails and flat peaks. The normal distribution has a kurtosis of 3, so any distribution with a kurtosis greater than 3 is considered leptokurtic, while any distribution with a kurtosis less than 3 is considered platykurtic.
Although the normal distribution is the most important special case, there are many other distributions that can be described as either leptokurtic or platykurtic. For example, the Cauchy distribution is leptokurtic, while the uniform distribution is platykurtic. Kurtosis is often used as a measure of how “peaky” a distribution is, but it
How to tell if your data is Leptokurtic or Platykurtic
One way to tell if your data is leptokurtic or platykurtic is to look at the kurtosis. Kurtosis is a measure of how peaked a distribution is. A leptokurtic distribution is more peaked than a normal distribution, while a platykurtic distribution is less peaked. You can calculate kurtosis using the formula: Kurtosis = (n(n+1)) / ((n-1)(n-2)(n-3)) * ∑((x_i – μ)^4) / σ^4 Where n is the number of data points, μ is the mean, and σ is the standard deviation. If Kurtosis > 3, then the distribution is leptokurtic. If Kurtosis < 3, then the distribution is platykurtic.If Kurtosis = 3, then the distribution is normal.
The benefits of having a Leptokurtic or Platykurtic dataset
There are several benefits to having a leptokurtic or platykurtic dataset. First, it can help to reduce the amount of noise in the data.
Second, it can improve the accuracy of estimates by reducing the variability of the data. Third, it can help to improve the interpretability of the results by making them more robust to outliers.
Finally, it can help to reduce computational costs by decreasing the number of data points that need to be processed. As a result, leptokurtic and platykurtic distributions can be very useful in statistical analysis.
How to adjust your data to make it more Leptokurtic or Platykurtic
One way to make data more leptokurtic is to add more observations at the extremes of the distribution. This can be done by collecting new data or by transforming the existing data. For example, if the data is normally distributed, it can be transformed into a log-normal distribution by taking the logarithm of each observation.
This will cause the extreme values to become more pronounced. Another way to make data more leptokurtic is to remove outliers. This can be done by applying a filtering process to the data or by using robust estimates that are less affected by outliers. Finally, leptokurtosis can also be increased by combining multiple distributions. This is often done in finance, where different asset classes are combined to create a more diversified portfolio.
When Leptokurtosis or Platykurtosis is a problem
When analyzing data, leptokurtosis and platykurtosis are two measures that are often used to describe the distribution of the data. Leptokurtosis is a measure of peakedness, and platykurtosis is a measure of flatness. If the data is well-behaved, then these measures will be close to zero. However, if there is a problem with the data, then one or both of these measures will be far from zero. For example, if the data is highly skewed, then leptokurtosis will bepositive and platykurtosis will be negative. In addition, if the data is multimodal, then leptokurtosis will be positive and platykurtosis will be positive. Knowing how to interpret these measures can be helpful in identifying problems with the data.
Examples of datasets that are either Leptokurtic or Platykurtic
There are many different types of data sets, and each has its own characteristics. Some data sets are leptokurtic, meaning that they have a high degree of kurtosis, or peakedness. Other data sets are platykurtic, meaning that they have a lower degree of kurtosis. Here are some examples of data sets that are either leptokurtic or platykurtic:
- Data from a survey of people’s income levels would likely be leptokurtic, as there would be a small number of people with very high incomes and a large number of people with relatively low incomes.
- Data from a study of the heights of people in a population would likely be platykurtic, as there would be a relatively even distribution of heights.
- The Data from a study of the ages of people in a population would likely be platykurtic, as there would be a relatively even distribution of ages.
- Data from a study of the weights of people in a population would likely be platykurtic, as there would be a relatively even distribution of weights.
Conclusion
In conclusion, it is important to understand the difference between leptokurtic and platykurtic distributions. Leptokurtic distributions are more peaked and have heavier tails than platykurtic distributions. Platykurtic distributions, on the other hand, are more flat and have lighter tails. This difference is important because it can affect the way that data is analyzed. Leptokurtic distributions are more likely to be affected by outliers, for example, while platykurtic distributions are less likely to be affected by outliers. Understanding the difference between these two types of distributions is essential for any data analyst.