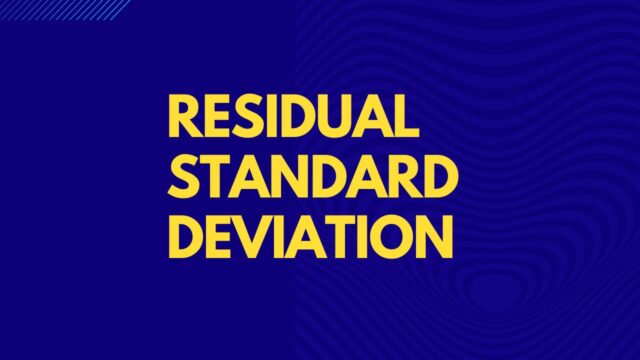
What is residual standard deviation and how is it used in statistics
In statistics, the residual standard deviation (RSS) is a measure of the variability of a data set that remains after accounting for the effects of other variables. It is calculated as the square root of the sum of the squares of the residuals (the difference between each data point and the mean). The RSS is often used to assess the goodness-of-fit of a statistical model. A lower RSS indicates a better fit, since it means that the model is able to explain more of the variability in the data. In some cases, the RSS can also be used to compare different models and choose the one that provides the best fit.
How to calculate residual standard deviation
To calculate RSD, one first needs to calculate the residuals, which are the differences between the actual data points and the predicted values. Once the residuals have been calculated, the RSD can be determined by taking the square root of the sum of squared residuals divided by the number of degrees of freedom. Although RSD is a relatively simple statistical measure, it can be very useful in understanding the variability of data.
Advantages and disadvantages of using residual standard deviation
There are both advantages and disadvantages to using this metric. One advantage is that it can be used to identify which factors are most important in explaining the variability in the data. However, a disadvantage is that it can be sensitive to outliers, which can lead to inaccurate results. In addition, the residual standard deviation can be affected by the sample size, which can make it difficult to compare results across different data sets. Overall, the residual standard deviation can be a useful tool for assessing data sets, but it is important to understand its limitations in order to avoid inaccuracies.
When is it appropriate to use residual standard deviation
RSD is typically used to compare different regression models and to select the best model for a particular data set. It can also be used to assess the goodness-of-fit of a regression model. When interpreting RSD, it is important to keep in mind that it will always be larger than the standard error of the estimate (SEE). This is because SEE only measures the variability of the estimates, while RSD also includes the variability of the actual values. Generally speaking, a smaller RSD value indicates a better fit.
How it is different from other measures of dispersion
There are a number of different measures of dispersion, or how spread out data points are. The most common measure is the standard deviation, which tells you how far away from the mean (average) each data point is. However, the standard deviation can be affected by outliers, or data points that are far away from the rest of the data. It is a measure of dispersion that is not affected by outliers. This makes it a more reliable measure when you want to get an accurate picture of how spread out your data is. However, it is important to note that the residual standard deviation is only accurate if your data is normally distributed. If your data is not normally distributed, then the standard deviation is a better measure of dispersion.
What sources of error can impact the results of using residual standard deviation?
When taking measurements, it is important to be aware of sources of error that could impact the results. One type of error is called residual standard deviation, which occurs when the measured value is different from the true value. There are several potential sources of this type of error, including human error, instrument error, and environmental conditions. To minimize residual standard deviation, it is important to take multiple measurements and to average the results. It is also important to use a high-quality instrument that has been calibrated correctly. By taking these precautions, it is possible to reduce the impact of residual standard deviation and obtain more accurate results.
How to avoid problems when using residual standard deviation in your statistical analysis
There are a few potential problems that can arise when using this in your statistical analysis. First, it is important to make sure that the residuals are normally distributed. If they are not, then the results of your analysis could be biased. Second, you need to be careful about outliers. Outliers can have a significant impact on this, and thus on the results of your analysis. Finally, it is important to use a consistent methodology when calculating the residual standard deviation. If you change the way you calculate it from one iteration to the next, then the results of your analysis could be inaccurate. By taking these precautions, you can avoid problems when using it in your statistical analysis.