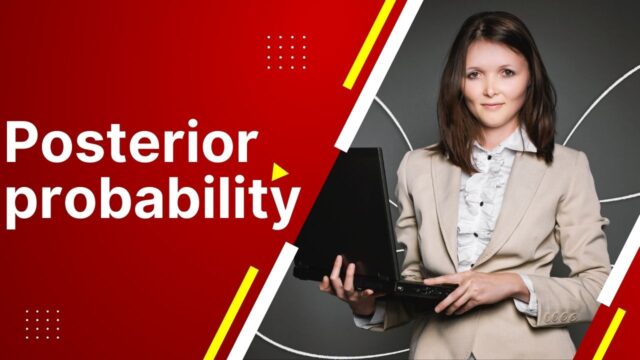
What is posterior probability and how is it different from other types of probability
Posterior probability is a type of probability that takes into account both the prior probability of an event and the evidence that has been collected about the event. For example, imagine that you are trying to decide whether or not to attend a concert. The prior probability is the likelihood that you will enjoy the concert without knowing anything about it. The posterior probability is the likelihood that you will enjoy the concert after taking into account all of the evidence that you have collected about it.
This evidence might include reviews from other concertgoers, information about the performers, and your own personal taste in music. In general, the posterior probability will be lower than the prior probability, because the evidence can both confirm and disconfirm our beliefs about an event. However, in some cases, the posterior probability can be higher than the prior probability, if the evidence is particularly strong.
How to calculate posterior probability
Bayes’ theorem is a formula used to calculate the conditional probability of an event, based on prior knowledge of conditions that might be related to the event. In other words, it allows you to update your estimate of the probability of an event occurring, based on new information. For example, imagine you’re trying to decide whether to buy a particular stock. You know that the stock has a 50% chance of going up and a 50% chance of going down, but you also hear that the company is about to release a new product that is expected to be very popular.
This new information will affect the probability of the stock going up or down, and Bayes’ theorem can be used to calculate the new probabilities. In this example, the posterior probability (the probability after taking into account the new information) would be higher than the prior probability (the probability before taking into account the new information). Bayes’ theorem can be used in many different situations where there is uncertainty about an event occurring, and it can be a valuable tool for making decisions in the face of new information.
Examples of how to use posterior probability
In statistics, posterior probability is the probability of an event occurring given that another event has already occurred. Bayes’ theorem is a way to calculate posterior probability. It states that the posterior probability of event A occurring is equal to the prior probability of event A multiplied by the likelihood of event A given that event B has occurred, divided by the prior probability of event B. In other words, posterior probability = (prior probability x likelihood) / prior probability of B. An example of how this might be used is if someone wanted to know the likelihood of it raining tomorrow given that the weather forecast says there is a 60% chance of rain.
The prior probability would be the percentage chance of rain without taking into account the forecast, which might be 50%. The likelihood would be the percentage chance of rain if the forecast is accurate, which would be 100%. using Bayes’ theorem, we can calculate that the posterior probability of it raining tomorrow given the forecast is (50% x 100%) / 60% = 83%. This means that there is an 83% chance of it raining tomorrow if the weather forecast is accurate.
Pros and cons of using posterior probability
The main advantage of using posterior probability is that it allows statisticians to take into account new information as it becomes available. This can be very useful in situations where data is constantly changing, such as in weather forecasting. The main disadvantage of using posterior probability is that it can sometimes be difficult to calculate. Another potential drawback is that, because it relies on data that has already been collected, posterior probability may be less accurate than prior probability in situations where the data is very limited or not representative of the population as a whole.
When is posterior probability the most useful?
Posterior probability is most useful when making predictions. For example, if you want to predict the likelihood of an event occurring, you can use posterior probability to calculate the chances. This is because posterior probability takes into account all of the available information. This includes both the evidence that supports the event happening and the evidence that goes against it. By considering all of this evidence, posterior probability can give you a more accurate prediction than if you only considered the evidence that supported the event happening.
In addition, posterior probability is also useful for making decisions. For example, if you are trying to decide whether or not to buy a lottery ticket, you can use posterior probability to calculate your chances of winning. This will help you to make a more informed decision about whether or not to purchase the ticket.