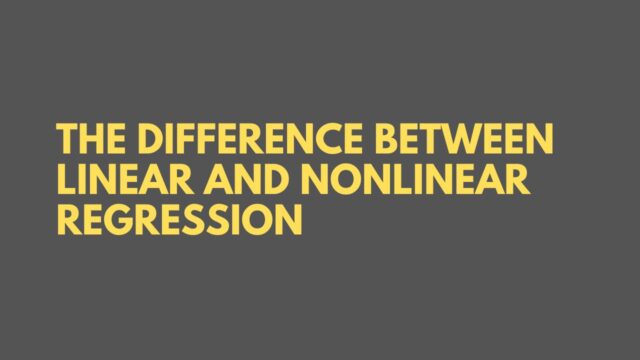
There are several differences between traditional linear and nonlinear regression. Classical assumptions are violated in nonlinear regression. In addition, the functional form is not linear, which violates the Gauss-Markov theorem. Nonlinear regression also violates the classical assumptions regarding dimensionality. This article will explain the difference between nonlinear and linear regression. You’ll also discover the benefits and disadvantages of each of the methods.
Transformable nonlinear models
Nonlinear regression models involve changing the variables in the model to fit the observations. These changes change the distribution of the errors and may affect the interpretation of the inferential results. The problem with nonlinear regression is that the nonlinear regression models can fail to converge and only be optimal locally. Here are a few things to keep in mind when working with nonlinear regression models. (Note that you may want to consider other approaches to data analysis as well.)
When using nonlinear regression, the variables in the model are the response variable, the function, and the parameters to be estimated. Each parameter can be tested for linearity. However, if the function is nonlinear, the second derivative with respect to the parameter will not be zero. Transformable nonlinear regression models have this unique feature. If this is the case, then you can use the model to combine nonlinear parameters within the given function.
A modern re-enactment of the experiment produces six data points. The input axis is the number of seconds in a second and the output axis is the distance traveled by the ball. By tuning these two parameters, you can find a linear relationship between the two features. A nonlinear graph is not as informative as a linear one. For example, a nonlinear function may have a linear relationship when regressed into the original nonlinear space.
Autocorrelated residuals
Autocorrelated residuals of nonlinear regression are errors that are not directly proportional to the data’s time series. This occurs when a regression model is fitted to a time series data set. Thus, the estimated model violates the assumption of no autocorrelation of errors. Such residuals are often considered to be inefficient and should be included in a model for better forecasts. This article explores the implications of autocorrelated residuals and the reasons why they appear.
Autocorrelation occurs when neighboring residuals are correlated. This is a sign of unobserved explanatory information that is not adequately described by the independent variables. Autocorrelation can occur in time-series models, which are particularly susceptible to this type of autocorrelation. Autocorrelation can be resolved by adjusting the model with independent variables that have time information. This autocorrelation may also be indicative of heteroscedasticity, or non-constant variance.
To overcome this problem, the model should incorporate time and distance as a factor. The t-test statistic should be higher for autoregressive models. The model must be adjusted to account for the dependence in order to achieve the best fit. In this case, a moving average or autoregressive model can be used. These models are better-fitting than traditional regression models. They also provide higher t-test statistics.
Cost of nonlinear regression models
In addition to the usual statistical techniques, nonlinear regression is very flexible, since it can fit a huge variety of shapes and curves. Instead of using simple addition and multiplication, nonlinear regression uses a more complex mathematical formula to represent the relationship between a response variable and its predictor. This means that nonlinear regression models are more suitable for modeling complex relationships involving time, population, and density.
However, the nonlinear regression model requires accurate specification of the relationship between the independent and dependent variables. Moreover, a poorly specified relationship may result in a no-convergent model. This is especially true when the dependent variable is a non-qualitative variable. Nonlinear regression models are much more expensive than their linear counterparts. However, they can fit an infinite number of functional forms. To calculate the cost of nonlinear regression, you must use a cost-benefit analysis to estimate the benefits and drawbacks of nonlinear regression models.
In a nonlinear regression model, the goal is to minimize the sum of squares. This measure tracks how far observations deviate from the nonlinear function. It is calculated by squaring each difference between the input variables and the data. The smaller the sum of squares, the better the model fits the data. Nonlinear regression uses a range of functions, such as logarithmic, exponential, power, Lorenz curves, and Gaussian functions.