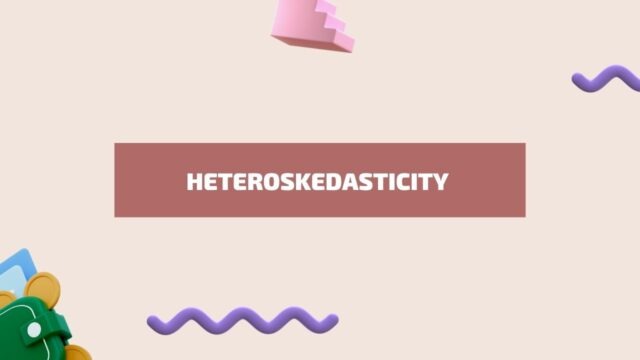
What is heteroskedasticity
Heteroskedasticity is a statistical concept that refers to a situation where the variance of a variable is not constant across all values of the variable. In other words, the variability of the variable changes as the value of the variable changes. This situation can occur when there is a relationship between the variable and another underlying variable. For example, heteroskedasticity might occur if there is a relationship between income and spending. Those with higher incomes would be more likely to have higher levels of spending, and thus their variance would be greater. Heteroskedasticity can also occur due to time-related effects, such as seasonal variation. It is important to take heteroskedasticity into account when analyzing data, as it can impact the results of statistical tests.
How to detect heteroskedasticity in data
There are a few different ways to detect heteroskedasticity in data. One method is to look at a scatterplot of the data. If the points are spread out unevenly, it may be an indication of heteroskedasticity. Another way to detect heteroskedasticity is to perform a statistical test known as a White test. This test compares the residuals from a regression model to see if they are equal across all values of the independent variable. If they are not, it is an indication of heteroskedasticity. Finally, many statistical software programs will automatically check for heteroskedasticity and report any findings in the output of a regression analysis. By understanding how to detect heteroskedasticity, statisticians can avoid drawing inaccurate conclusions from their data.
Causes of heteroskedasticity
There are a number of factors that can cause heteroskedasticity, including outliers, non-normal distributions, and periodicity. In some cases, heteroskedasticity can be due to simple randomness. However, it can also be caused by factors such as incorrect data entry or incorrect model specification. When heteroskedasticity is present, it can lead to inaccurate statistical inferences. For this reason, it is important to be aware of the potential causes of heteroskedasticity and to take steps to avoid them.
How to deal with heteroskedasticity in data analysis
Heteroskedasticity is a common problem in data analysis, in which the variance of a dependent variable is not constant across different levels of an independent variable. This can lead to inaccurate results and invalid conclusions. There are several ways to deal with heteroskedasticity, including transforming the data, using weighted least squares regression, or adding a dummy variable. In some cases, it may also be possible to use parametric methods such as GARCH or ARCH. The best approach depends on the specific data and the goals of the analysis. However, heteroskedasticity is a problem that can be dealt with effectively if it is anticipated and taken into account from the start.
Examples of heteroskedasticity
There are many examples of heteroskedasticity in the real world. For instance, consider a situation where people’s incomes vary widely. In this case, we would expect that people with higher incomes would tend to spend more on luxury items than those with lower incomes. As a result, the demand for luxury goods would be more volatile than the demand for necessities.
Another example of heteroskedasticity can be found in the stock market. Investors’ confidence tends to fluctuate, which leads to wide swings in stock prices. This volatility can make it difficult to predict how the market will behave in the short-term. However, over the long-term, the market is much more stable. These are just a few examples of heteroskedasticity that illustrate how this concept can have a real-world impact.