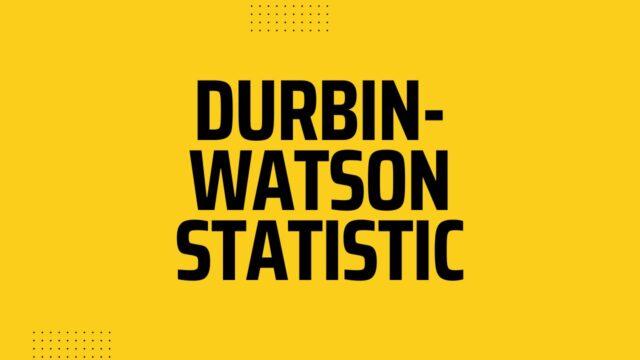
What Is the Durbin-Watson Statistic?
The Durbin-Watson statistic is a statistical test for autocorrelation. It was named for Geoffrey Watson and James Durbin. John von Neumann developed the small sample distribution for this statistic. This article will explain its definition and limitations. In addition, you’ll learn how to apply it to your own research. Here are some practical examples. Listed below are some examples of applications of this statistic.
Test statistic
The Durbin Watson statistic is a test statistic used to detect the presence of autocorrelation. It is named for its discoverers, James Durbin and Geoffrey Watson. John von Neumann first derived its small sample distribution and its symmetric properties. However, some recent developments have changed the way this statistic is used. Nevertheless, it remains a popular statistical tool. Learn how to use it to analyze the autocorrelation of your data.
The Durbin-Watson statistic ranges from 0 to 4, and is always between 0 and 4. This value less than 2 may indicate positive serial correlation, while a value of two or more indicates negative serial correlation. Field (2009) recommends a value of d of less than 1.5 as a sign of concern. The same rule applies to other statistical tests if the d is greater than two. But remember that the higher the d, the less likely the autocorrelation is.
Range of values
The Range of Values for Durbin Watson Statistics is used to assess the presence or absence of autocorrelation between residuals in a data set. This statistic takes values between 0 and 4. Values below 2 indicate no autocorrelation and values above 4 indicate negative autocorrelation. The Durbin-Watson statistic is commonly referred to as BLUS, and reflects the best linear unbias scalar covariance matrix.
The Range of Values for Durbin-Watson Statistic is a key feature to remember when evaluating data. The Durbin-Watson Table contains critical values, lower and upper limits, sample size and the number of variables used in the statistical model. This table also indicates the significance of the statistic based on its values for k, n and alpha. If the Durbin-Watson statistic is below 1.5, it may indicate that autocorrelation is present in the data set. On the other hand, if the DW statistic is above 2.5, this indicates a low-level positive autocorrelation.
Validity
The validity of a statistical test can be determined by examining the distribution of a sample. The Durbin-Watson statistic is a popular option for estimating the likelihood of a given outcome. The standard N (one, zero) distribution performs well in this scenario, and a tanh adjusted Durbin-Watson estimate, also known as TADW, eliminates this problem.
The Durbin-Watson statistic detects autocorrelation in residuals. A value of 0 indicates no autocorrelation, and a value of two to four implies that there is. For stock price analysis, the DW statistic is an important tool in predicting stock price movement. To use this statistic, you must ensure that the sample size is large enough to detect all trends. The DW statistic is highly significant for both small and large sample sizes.
Limitations
The limitations of the Durbin Watson statistic include a lack of inconsistency. As the name suggests, this statistic looks at a series of regressors and determines whether the values fall within a normal range. In most cases, these boundaries are within a reasonable range. Generally, the lower bound is less important than the upper bound, which is a concern when one is considering correlation.
The value of the Durbin Watson statistic varies from 0 to 4, with values between 0 and 2 indicating positive or negative autocorrelation. Although exact critical values are difficult to determine, significance tables may be used to help make a decision. These tables include sample size, number of regression parameters, and upper and lower bounds for the statistical significance. Although these tables are not perfect, they can provide a useful guideline for interpreting the data.
Uses
The Durbin-Watson statistic is used to measure serial correlation in regression. Many regression analysis programs display this statistic. Some of these programs use the Cochrane-Orcutt procedure to correct the statistic. However, the Durbin-Watson statistic is not applicable in some situations. The likelihood ratio test can be used in large samples. The SAS program has a Durbin-Watson option in the Proc Reg.
The Durbin-Watson test is most commonly used to determine whether residuals are autocorrelated. However, it can also be used to test whether two or more independent variables have the same effect on the sample’s autocorrelation. For example, if the autocorrelation coefficient is greater than zero, the Durbin-Watson test can detect this and provide a significant result. However, if the autocorrelation coefficient is lower than 0.5, the Durbin-Watson test is not significant.