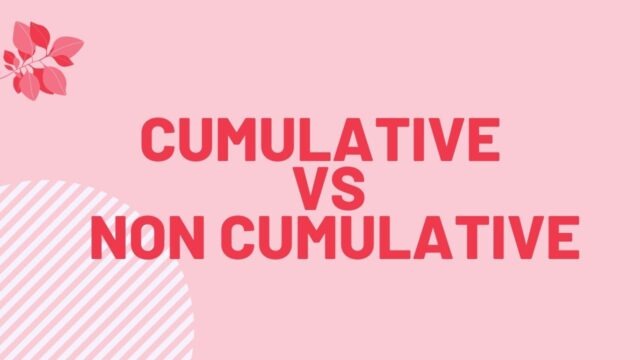
When it comes to data, there are two main types of distributions: cumulative and non-cumulative. Cumulative distributions are those in which the total sum of all values is always known, while non-cumulative distributions involve a rolling total that can change with each new data point. In this article, we’ll explore the differences between these two types of distributions and when you might want to use one over the other.
Additional reading:
Cumulative vs Non Cumulative
One of the most important aspects of any investment is whether it is cumulative or non-cumulative. Cumulative investments are those where the interest earned is added to the principal amount, so that the next period’s interest is earned on both the original principal and on the accumulated interest. Non-cumulative investments, on the other hand, do not have this compounding effect, so interest is only earned on the original principal. Cumulative investments typically offer higher returns than non-cumulative ones, but they also involve more risk. As a result, it is important to carefully consider your goals and risk tolerance before deciding which type of investment is right for you.
What is cumulative inflation and how does it work
Cumulative inflation is the total amount of inflation that has occurred over a period of time. Non-cumulative inflation, on the other hand, is the rate of inflation that occurs in a single year. In order to calculate cumulative inflation, economists add up the rates of inflation for each year and then multiply the total by the number of years in the period. For example, if there was an annual inflation rate of 2% over a 10-year period, the cumulative inflation would be 20%. Cumulative inflation can have a significant impact on purchasing power and economic growth.
For instance, if prices are rising at a rate of 5% per year, it would take $100 to purchase goods that cost $95 the previous year. Cumulative inflation can also make it difficult to compare prices across different periods of time. For example, a good that cost $10 in 1990 would cost $28.50 in 2019, an increase of 185%. Cumulative inflation erodes the value of money over time and can have a profound impact on economies.
What are the benefits of cumulative interest
Interest is the price of money, which is the percentage charged on loans or earned on deposits over a period of time. Cumulative interest is the total interest that accrues on an investment or loan over time. Non-cumulative interest accrues each period but is not compounded, so it doesn’t build up over time. The main benefit of cumulative interest is that it can compound, or grow, at a faster rate than non-cumulative interest.
Compounding occurs when interest is reinvested back into the principal, so that the interest earned in one period also earns interest in the next period. This can cause Cumulative Interest to grow at an exponential rate. For example, if you have a $1,000 loan with 10% annual interest and make no payments for 5 years, the total Cumulative Interest will be $2,592.86. In contrast, if the interest were non-cumulative, it would only be $500 after 5 years ($1,000 x 0.10 x 5). This example demonstrates how Cumulative Interest can quickly add up over time. Thus, Cumulative Interest can be extremely beneficial for long-term investments and savings plans. However, it’s important to remember that
How to calculate cumulative return on investment
Cumulative return on investment (CROI) is a measure of the performance of an investment over its lifespan. Unlike non-cumulative return on investment (NCROI), which simply looks at the difference between the original investment value and the current value, CROI takes into account all of the cash inflows and outflows that have occurred over the life of the investment. As such, it provides a more holistic view of an investment’s performance. To calculate CROI, simply divide the total value of all cash inflows by the total value of all cash outflows. This will give you a percentage that represents the Cumulative Return on Investment. For example, if an investment has generated $5,000 in cash inflows and $4,000 in cash outflows over its lifetime, the Cumulative Return on Investment would be 25%.
What is the difference between a cumulative and non-cumulative distribution
Cumulative distribution functions (CDFs) and non-cumulative distribution functions (non-CDFs) are often confused because they both describe the same underlying data. The difference lies in how the information is presented. A CDF shows how much of the data is less than or equal to a certain value, while a non-CDF shows how much of the data is greater than a certain value. Cumulative distribution functions are typically used when we want to know the probability that a random variable will take on a value less than or equal to x. Non-cumulative distribution functions areused when we want to know the probability that a random variable will take on a value greater than x. In general, CDFs are used more often than non-CDFs because they provide more insight into the data. However, there are some situations where a non-CDF is more appropriate, such as when we’re interested in tail probabilities.
Examples of when to use cumulative and non-cumulative distributions
Cumulative and non-cumulative distributions are two types of ways to look at data. Cumulative distribution function (CDF) is a graphical representation of the probability that a given outcome will occur or be exceeded. Non-cumulative distribution function is a graphical representation of the likelihood that an outcome will occur. In general, cumulative distribution functions are used when one wants to calculate the probability of an event occurring, while non-cumulative distribution functions are used when one wants to calculate the likelihood of an event occurring.
There are several examples of when each type of distribution would be used. For instance, if someone were flipping a coin, they would use a cumulative distribution function to calculate the probability that the coin would land on heads at least once in four flips. However, if they were interested in calculating the likelihood that the coin would land on heads exactly three times in four flips, they would use a non-cumulative distribution function.
Cumulative and non-cumulative distributions are two important tools for understanding data. By understanding when to use each type of distribution, one can more accurately interpret information.
Advantages and disadvantages of cumulative and non-cumulative distributions
Cumulative distribution functions (CDFs) and non-cumulative distribution functions (PDFs) both have their own advantages and disadvantages that need to be considered when making decisions. Cumulative distribution functions are often used when it is important to know how much of a given quantity is less than or equal to a certain value. For example, if you wanted to know what percentage of people in a population are shorter than 5 feet tall, you could use a CDF. On the other hand, non-cumulative distribution functions are more commonly used when it is important to know how likely it is that a given value will occur. For example, if you wanted to know the probability that it will rain tomorrow, you would use a PDF. Each type of distribution has its own strengths and weaknesses, so it is important to choose the one that is best suited for your needs.
When to use a cumulative distribution function over a non-cumulative distribution function
Cumulative distribution functions (CDFs) and non-cumulative distribution functions (non-CDFs) are both types of statistical models that can be used to represent data sets. CDFs are often used when it is desirable to know the likelihood of observing a value that is less than or equal to a certain quantity, while non-CDFs are more useful for determining the likelihood of observing a value that is greater than or equal to a certain quantity. In general, CDFs are preferable to non-CDFs whenever it is important to know the probability of observing values that fall below (or above) a certain threshold. For example, CDFs would be more appropriate than non-CDFs for data sets that represent exam scores, since it is usually more important to know the probability of getting a score below (or above) a certain cutoff.
However, non-CDFs would be more appropriate for data sets that represent income levels, since it is usually more important to know the probability of making more (or less) than a certain amount of money. Cumulative distribution functions can also be used when it is necessary to model data with very small probabilities, such as data from radioactive decay experiments.