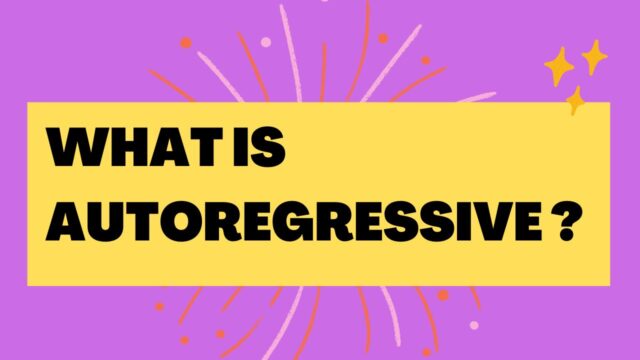
Important Things You Should Know About Autoregressive Models
Autoregressive models are models that use random processes to describe time-varying phenomena. In the context of economics and nature, they are often used to explain financial markets and the price movements that accompany them. There is a great deal of controversy surrounding this model and its predictive ability, but it remains an important tool for economists. Listed below are some of the most important things you should know about autoregressive models. They may seem complicated, but they can help you make better decisions about your financial future.
Variations of autoregressive processes
Statistical analysis of stock prices has been an important application of Autoregressive models. These methods forecast arbitrary numbers of periods by using the first period for which data are not available. The first period is used as the generating variable for the process, and the value of i is substituted by the previous observed values of i. The error term is set equal to zero. The output is the forecast for the first unobserved period.
The AR(1) and AR(2) autoregressive processes have two different types of dependence. The AR(1) is based on the value immediately preceding it, while the AR(2) is based on two previous values. The AR(0) autoregressive process is used when the values are non-correlated and there is no dependence between terms. The coefficients are calculated using the least-squares method. However, the difference between the two types is significant.
The second type of autoregressive model is nonstationary and has a unit root. When the coefficients are in the triangle, it is stable. When the roots are outside the unit circle, the process is non-stationary. If the coefficients are inside the unit circle, it is stable. The p-lag model is also supported in PyMC3.
Uncertainty about autoregressive coefficients
The uncertainty about autoregressive coefficients in a logistic growth model can be evaluated using a Dickey-Fuller test. This test is used to determine whether the autoregressive time series errors have a unit root, stochastic trend, or stationarity. It is typically performed using the usual OLS (t)-statistic. The following sections describe two ways to calculate the uncertainty about autoregressive coefficients in a logistic growth model.
An autoregressive model can forecast arbitrary number of periods. When the forecasted value is lagged in the right side of the equation, the predicted value is used in place of the actual data for that period. If the forecasted value is larger than the expected value, more lagged values will be used to construct the future model. Uncertainty about autoregressive coefficients is one of the most common problems faced in statistical analysis.
Predictive performance of autoregressive models
When evaluating the predictive performance of an autoregressive model, you can compare its predictions to test data. Autoregression uses a combination of estimation and out-of-sample testing. Afterward, more data may become available and you can evaluate the predictive performance again. Once you have evaluated the model’s predictive performance, you can make improvements if necessary. To understand this better, consider the following example.
Technical analysts often use autoregressive models to forecast stock prices. Since the models rely on past data, they implicitly assume that fundamental forces will not change. However, when these forces do change, the model’s predictions can no longer match the market’s reality. In fact, as a result, traders have continued to refine their methods. One example is the Autoregressive Integrated Moving Average. This type of model takes into account market trends, cycles, seasonality, and errors.
The use of ARMs is a relatively new field. This type of model has many applications, ranging from minute-by-minute prediction to the detection of irregular heartbeats. This type of model has several advantages. In addition to minute-by-minute prediction, it can be used for various applications, such as the analysis of response to training and detecting arrhythmias. So, if you are interested in improving the accuracy of your heart rate prediction, this model might be a good choice for you.