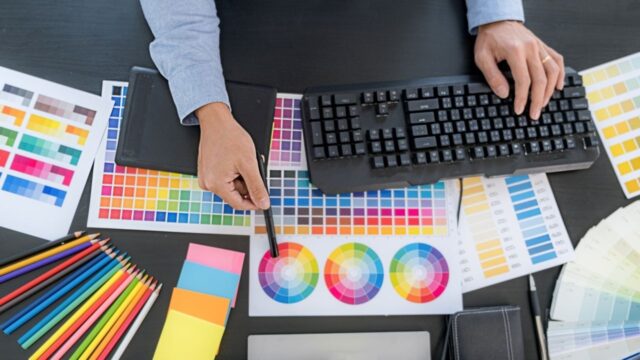
In the world of research and statistics, two types of errors are commonly encountered: alpha error and beta error. Understanding the difference between them is essential for researchers who wish to produce reliable and valid results. Alpha error, also known as type I error, occurs when a statistical test incorrectly rejects the null hypothesis, while beta error, or type II error, occurs when a test fails to reject a false null hypothesis. In this blog post, we will explore the differences between alpha error and beta error in detail, and provide examples to help clarify the concepts.
Alpha Error
Alpha error occurs when a researcher concludes that there is a significant difference between two groups or variables when no real difference exists. This type of error usually stems from a small sample size, poor data quality, or using an inappropriate statistical test that produces a high rate of false positives.
As a result, researchers may reject the null hypothesis when it is actually true, leading to a false conclusion that the study variables are related. For example, if a drug trial is conducted with too few participants, and it is found to have a significant effect on reducing symptoms, the researchers may make the conclusion that the drug is effective. However, this conclusion may be incorrect if there were not enough participants for the results to be statistically meaningful.
Beta Error
Beta error occurs when a researcher fails to reject a false null hypothesis, leading to a conclusion that there is no significant difference when there actually is. Beta error is commonly linked to a low rate of statistical significance, or the use of underpowered statistical tests.
For example, if a researcher conducts a test to identify whether a new drug has an effect, and the test fails to identify a difference with a p-value greater than .05, the researcher may falsely conclude that there is no effect. However, if they had used a more sensitive test with a smaller p-value, they may have found evidence of a significant impact.
Factors That Influence Error Rates
Both alpha and beta errors are influenced by various factors, including the level of statistical significance used, the sample size, and the statistical test used.
The level of significance determines the likelihood of rejecting the null hypothesis when it is true (alpha error) and the likelihood of failing to reject it when it is false (beta error).
A small sample size increases the likelihood of both alpha and beta errors, as there is more variability in the data and less precision in the results. Finally, the statistical test used plays a significant role in determining the error rate, as some tests are more sensitive and specific than others.
Minimizing Error Rates
To minimize both types of errors, researchers should conduct appropriate sample sizes, use efficient and sensitive statistical tests, and adjust the level of statistical significance according to the research question and available data. Additionally, replication studies can help to verify initial findings and increase confidence in results. Finally, pre-registration of studies and publication of results can promote transparency and reduce selective reporting, which can contribute to inflated error rates.
Conclusion:
In conclusion, alpha and beta errors are crucial concepts in the world of statistics and research. Understanding the differences between them is essential for producing reliable results and avoiding false conclusions. By appropriately selecting statistical tests, adjusting significance levels, and conducting larger sample sizes, researchers can minimize the likelihood of both alpha and beta errors and increase confidence in their findings.